
In this paper, we introduce a uniform framework called the quantum pixel representation (QPIXL) that overarches all previously mentioned quantum image representations and probably many more. Second, image processing operations can be performed simultaneously on all pixels in the image by exploiting the superposition phenomenon of quantum mechanics. First, their flexibility in encoding the positions and colors in a normalized quantum state.
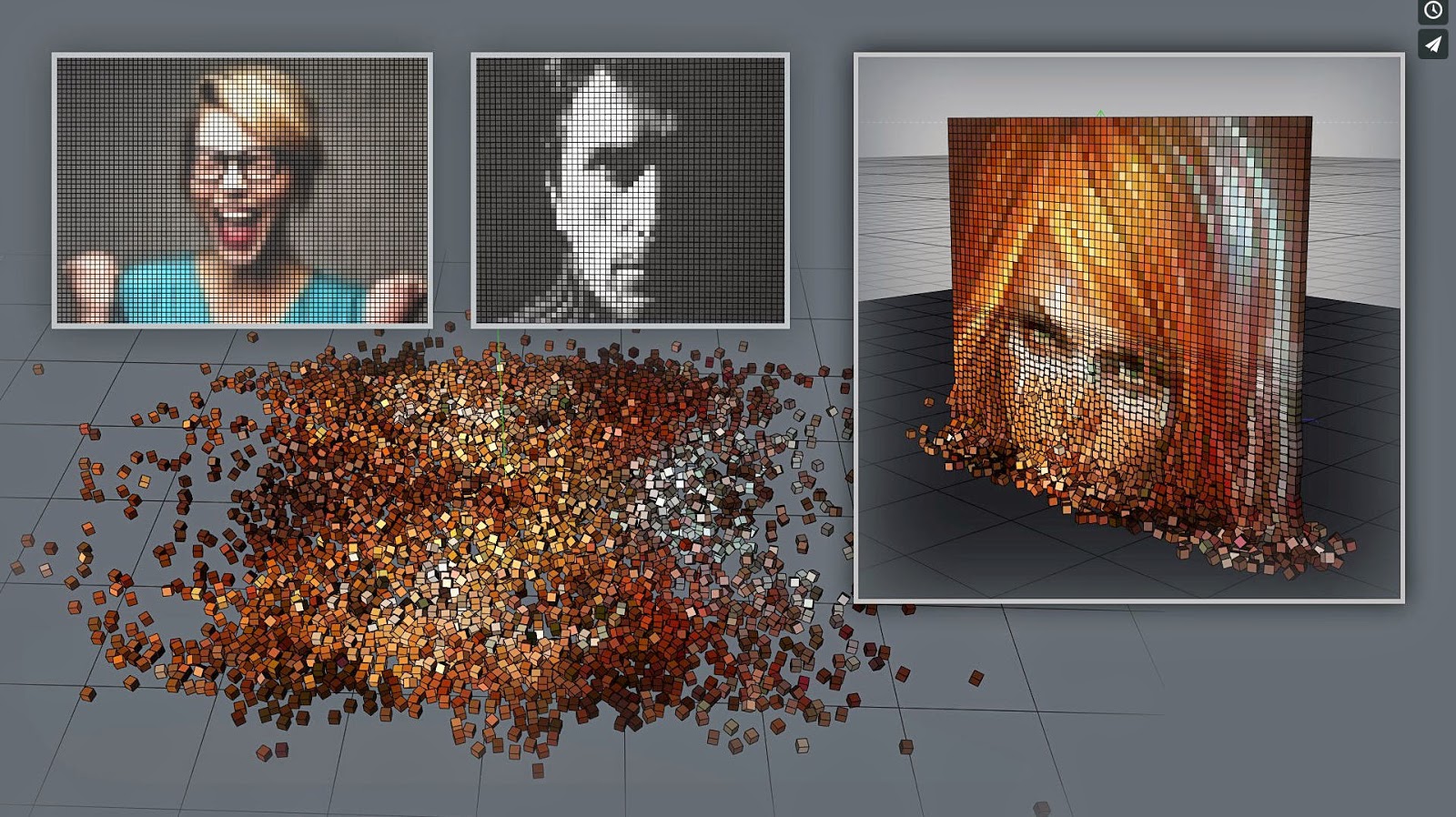
These QIR methods became extremely popular due to two main factors. The flexible representation of quantum images (FRQI) 5, 6, the improved flexible representation of quantum images (IFRQI) 7, the novel enhanced quantum representation (NEQR) 8, the improved novel enhanced quantum representation (INEQR) 9, the multi-channel representation of quantum images (MCRQI/MCQI) 10, 11, the novel quantum representation of color digital images (NCQI) 12, and the improved novel quantum representation of color digital images (INCQI) 13 are among the most powerful existing QIR methods. A variety of quantum image representation (QIR) methods has been developed 4. QIMP algorithms are used on images that have been represented in a quantum state.
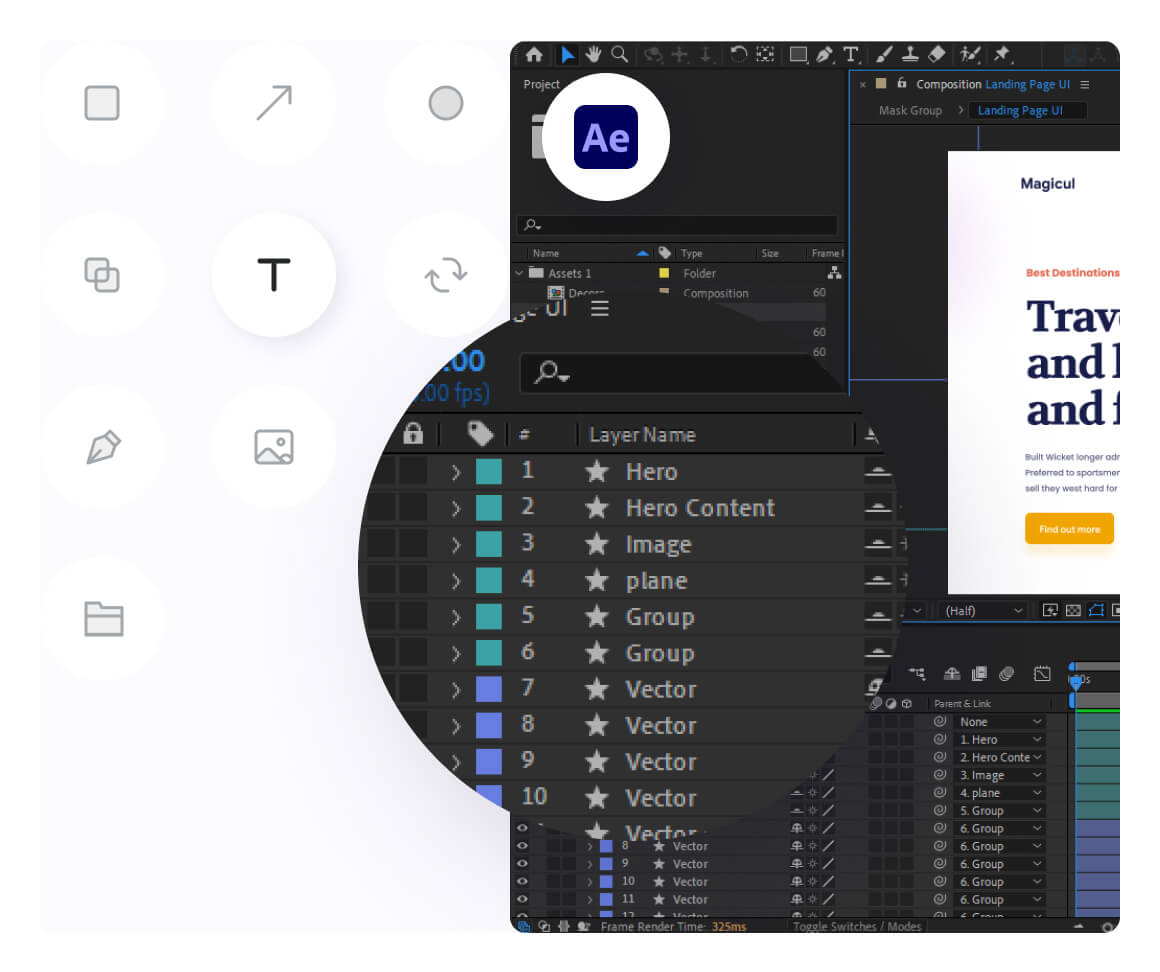
Quantum image processing (QIMP) extends the classical image processing operations to the quantum computing framework 3. Hence, optimizing quantum circuits into short-depth circuits is extremely important to successfully produce high-fidelity results on NISQ devices. The current and near-term quantum computers, known as noisy intermediate-scale quantum (NISQ) devices, are characterized by low qubit counts, high gate error rates, and suffer from short qubit decoherence times 2. One of the research challenges to make quantum computing a viable platform in the post-Moore era is to reduce the complexity of a quantum circuit to accommodate many qubits. Quantum computing holds the promise of speeding up computations in a wide variety of fields 1, including image processing. Consequently, finding more efficient ways of handling scientific data is an important research priority. More specifically, the analysis of image-based data becomes increasingly challenging using current classical algorithms.

The growth in scientific data size and heterogeneity overwhelms current statistical and learning approaches for analysis and understanding.
